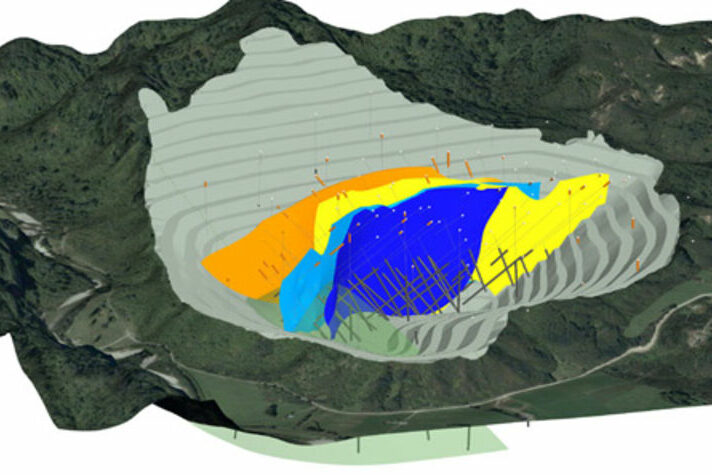
Machine Learning in Geology
Machine learning in Geology is being used for various applications and in all stages of the mining cycle. These include exploration, mine geology, resource estimation, mine planning, mineral processing, tailings management, and metallurgy. There is an increased interest in machine learning and artificial intelligence, in large part due to the data revolution.
The adoption of these technologies depends on the availability of data and the agility of individual companies.
What is Machine Learning?
Machine learning (ML) is a subdiscipline of artificial intelligence (AI) that is concerned with computer systems’ ability to automatically learn and improve from experience without being explicitly programmed. In mining and resource geology, machine learning focuses on the development of algorithms and their applications (e.g. ‘programs’) that can access data and use it to learn for themselves.
Components of Machine Learning
Data, algorithms and computing power are key elements that enable machine learning applications. There is a lot of variability in the type and characteristics of data by discipline and within any discipline. To leverage machine learning and related technologies requires both specific industry expertise and expertise in data science.
Machine Learning in Geology and Geosciences
In geosciences, qualitative data in the form of field observations to thin section descriptions are common place. The cost of this type of data is also quite high per data point, as the sampling, processing and analysis stages are time consuming and require extensive subject matter expertise and labour. Quantitative data also exists in geoscience in the form of chemistry and physical properties, and geophysical survey data.
The value added by Machine Learning
ML is able to make use of this large variety of data for algorithm training, in order to look for patterns in data and extract insights such as high-dimensional, nonlinear and spatially localized relationships.
The relationships are modelled by one or more algorithms, which is then used in an inductive manner to make predictions.
This process is highly automatable and with the advancement of computing power, is able to deal with large amounts of data in real-time.
Machine learning applications in Geology
A trained ML algorithm can be deployed to perform a range of tasks, such as processing live sensor data (e.g. in online monitoring situations) or to perform resource prediction (e.g. using geological and geochemical data).
Although the discipline has been in existence for over 7 decades, application in mining geology only recently gained momentum. ML bridges the gap between traditional qualitative/observational geology and quantitative computational modelling, and will soon become an area of specialisation in the field of applied and theoretical geology.
Machine Learning in Mining Geology
In mining geology, machine learning can be applied in areas such as
- local and regional mapping,
- anomaly detection,
- ore characterisation,
- target exploration,
- resource definition,
- geological and structural domaining, and
- facies classification.
Machine Learning for Resource Geology and Estimation
In mineral resources geology, ML has many applications such as
- incorporation of descriptive geological data and chemical assays for statistical domaining,
- in-situ grade prediction for grade control,
- resource estimation and classification,
- outlier detection,
- risk assessment
- a quicker and reliable method to model complex ore bodies.
In theoretical geology, ML can readily assess large amounts of data for the purpose of hypothesis testing.
Leveraging Data
It is no secret that the collection of mineral resources data is an expensive task and requires high diligence in quality assurance (QA) and quality control (QC). Today’s business world is driven by data, especially Big Data, and the minerals industry is ripe with legacy data.
Legacy data from every stage of the mining life cycle, such as exploration, mapping, assay, operation and monitoring are abundant but stored in various data silos, some of which are unfortunately inaccessible, lost or unintelligible due to historical data management practices.
Solving Problems in Mining with Machine Learning
A key challenge in mining and resource geology has been to adequately supply an increasing demand of raw materials, while facing a decrease in typical ore grades and other complicating challenges as we collectively look farther and deeper for resources.
Among the plethora of solutions to this system-level challenge, technology is one key enabling pillar among a few key ones for the continued supply of raw materials.
The aim of machine learning in mining and resource geology is to enhance, via a combination of
- increase in precision,
- reduction in uncertainty and
- increase in automation in every stage of the mining cycle, from the identification and characterization of ore deposits,
- prediction of in-situ grade and quantity, and
- classification of ores based on their intrinsic characteristics for the purpose of exploration, mining and mineral processing.
Conclusion
In conclusion; incorporation of machine learning in mining and resource geology comes with many benefits. For these benefits to be realised successfully, requires subject matter expertise. Machine learning is a tool based on computer language. As an analogy, knowing the English language perfectly does not mean you can write a good poem or a good book.
In very much the same way, effective solutions in for machine learning in geology and mining will require a subject matter expert who understands the problem and nuances in the field of concern, and can translate it across fields from one scientific field into the field of data science to yield solutions that address relevant problems. That can be either in the form of an individual with expertise in both data science and the field in question, or a multi-disciplinary team working in collaboration and with guidance from the multi-disciplinary subject matter expert when necessary.
See Next: Where machine learning can be used in mineral projects
For any enquiries, contact us at SmartMin or fill in our online contact form
No Comments